Imagine you're a Quality Control manager, and your job is to maintain the cleanliness of a cleanroom. For this, you collect data about the site: you layout settle plates, place contact plates, conduct finger dabs, and take air samples, and you keep records of all this data.
This data-collection process becomes more sophisticated as time goes by; you record which operator worked in which room and when, and store this in the database. This information is known as metadata: data about the data.
Next, you collect more metadata, this time about the conditions of the room itself: you record the temperature, humidity and air pressure, measured at various intervals throughout the day. This information is stored in a spreadsheet or database so that it's easy to access, and you believe you have an accurate picture of what's going on in your cleanrooms.
You also have the task of drawing out insights from the data. But where to begin? Your database is so vast it's dizzying. You're drowning in data.
The DIKUW hierarchy
Before we go any further, we want to make one thing clear: this article is not going to tell you what to do with your data. That would be impossible; we don't know any particulars about your facility, or what it is you're trying to achieve. Instead, we'll show you how to use the DIKUW hierarchy: Data, Information, Knowledge, Understanding, Wisdom.
The DIKUW is a framework that we've found invaluable for thinking about data and generating useful insights. It isn't a prescriptive guide, but an outline of a typical data journey. It will help you see where you are in your data journey, and what to expect. It will also help you understand the limitation of data.
Systems theorist Russell Ackoff outlined the DIKUW hierarchy in his paper "From Data to Wisdom" in 1989, and described it as a 5-step process, which we all go through as individuals and institutions, of transforming data into wisdom.
Step 1: The data
Data is symbols we use to represent information about the world. It might be the number of growths on a plate of agar jelly, or the temperature of a room five-minute intervals. It might be recorded by a human, putting ticks on a page, or by a machine. So long as its symbols are representing something of interest in your cleanroom, it counts as data.
In its raw form, data is often very difficult for a human to read. Imagine a list of ten-thousand numbers, each between zero and one and rounded to ten decimal places. A human, looking at these numbers, is unlikely to be able to glean much of use from it. Data must be transformed to become useful.
Data that is improperly recorded and stored will be useless in helping you understand your cleanroom
Data integrity is also worth mentioning here. Data that is improperly recorded and stored will be useless in helping you understand your cleanroom. Worse, it might lead you to believe things that aren't true. If you can't ensure the integrity of your data, then there's no point moving on to step 2 in this process.
Let's introduce a running example to highlight the differences in the levels of the hierarchy: collecting data might be counting the number of grows of CFUs on settle plates in a cleanroom and recording them in a database.Step 2: Transforming data into information
Information is processed data. This processing aims to make the data transparent to a human. It's normally aimed at answering the who, what, when, and how many.This process might be achieved in many ways. For example, the ten-thousand numbers mentioned above could be averaged to produce a single number, if that's appropriate. The point is not that averaging is the most useful summary, only that it's one possible way.
Already we see the application of human judgement into this process. There is no correct way to transform data into information, only better or worse ways, depending on your goal. Experience, as much as rational thought, will teach you the best way to proceed here.
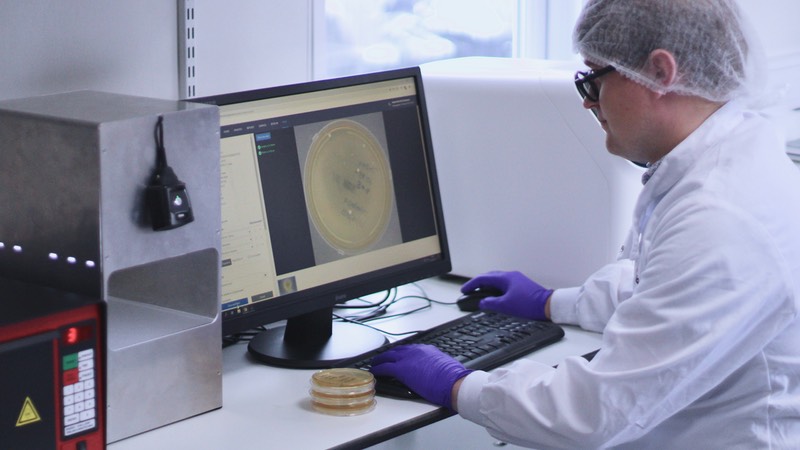
The usual way to store this information is in relational databases. The canonical version of these databases is spreadsheets. The rows always represent instances (or tuples), and the columns represent the attributes of these instances. For example, in one column of a database, you might record how many CFUs were present in a particular session, and in another, you might record metadata: which operator was present in the room. Notice that this allows an investigator to draw out the relationships between the data. In this way, it's useful to think of information as being refined data plus relationships.
Information can also be drawn out of data through visualisation tools. The investigator has a whole host of tools, from graphs and bar-charts to cluster-plots and histograms. Human judgement must also be exercised here: the best way to display the data might depend on factors such as data complexity and the technical know-how of the audience. The purpose of these tools is always to make it easier for a human to understand the data.
Back to our CFUs counting example, the data we've recovered might be plotted on a graph against time to show that the numbers of CFUs have increased over time.
Step 3: From information to knowledge
To gain knowledge means to spot patterns in the information and learn from them. When you're asking questions like "how might we reduce the number of CFUs in the air in a cleanroom?" you're attempting to gain knowledge. In this case, you might believe that the air filters are not working properly, so you replace them, and record data based on the new air samples. If the number of CFUs goes down, you can say you know something about air samples in your facility.
Knowledge is a key component in problem-solving. The more you know about your cleanroom, the more power you have over it. Notice also the importance of contextual information here. Knowledge is not something that can be obtained simply by processing the data. You require specialist domain knowledge to be able to interpret the information and make it useful.
You might employ statistical or machine learning methods to your data to draw out the deep relationships between the variables you're measuring
At this point, you might employ statistical or machine learning methods to your data to draw out the deep relationships between the variables you're measuring, which cannot be detected by eye. Machine learning tools, along with the domain-specific knowledge of an expert, can reveal a great deal about your cleanroom that would otherwise be invisible.
Continuing our example, through machine learning techniques and domain-specific knowledge, you might have noticed that there's a seasonal trend in the number of CFU recoveries: it tends to be higher during some times of the year, and lower in others.
Step 4: Obtaining understanding from knowledge
Understanding means to grasp the fundamental principles that govern a system. It connects different pieces of knowledge to draw a single, consistent picture. It might be understanding that human error will always be a major cause of breaches in your facility and that steps must always be taken to reduce its impact. Or it might be understanding that changes in the environment around your facility are causing the appearance of novel CFUs.
Understanding answers "why" questions such as why is one filter more appropriate than another? Why are more breaches occurring on a Monday morning than a Tuesday afternoon?
Again, the use of machine learning and statistical techniques can help you understand more about your facility. Success in that relies on the quality of the questions asked. Machine learning by itself will not help you understand your facility better, but it can be a powerful tool in building your understanding.
Machine learning by itself will not help you understand your facility better, but it can be a powerful tool in building your understanding
In our example, as you know that the fields around your facility are causing spikes in the number of CFUs over time, you decide to build your next facility in a location that does not have fields close by
Step 5: Wisdom
If understanding is the ultimate refinement of knowledge, then where does wisdom come into this? Wisdom is the addition of human value judgements in the process. It helps answer the "should we" questions.
The method of turning data into understanding pertains to the gradual increase of efficiency of a task. The more completely you understand the forces at work in your cleanroom, the more likely you are to keep it to the required cleanliness. But understanding how your cleanroom works is not going to select which task you ought to perform next.
In our example, as you know that the fields around your facility are causing spikes in the number of CFUs over time, you decide to relocate your facility to a location that does not have fields close by.
In our day-to-day business at Microgenetics, we use the framework outlined in this article to inform our decisions on how we design our products. Each step poses its own unique problems. Data must be accurately recorded and maintained properly.
The movement from data to information must be as painless as possible, and tools must be flexible. Statistical inference and machine learning can be used alongside domain expertise to build knowledge and understanding, but only where those tools yield interpretable outputs. Tools like these do exist, such as Microgenetics’ SmartControl for capturing and analysing microenvironmental monitoring data, and can lead to more effective decision-making and ultimately a higher state of control.
N.B. This article is featured in the March 2020 issue of Cleanroom Technology. Subscribe today and get your print copy!
The latest digital edition is available online.